PAIP 2023: Tumor cellularity prediction in pancreatic cancer (supervised learning) and colon cancer (transfer learning)
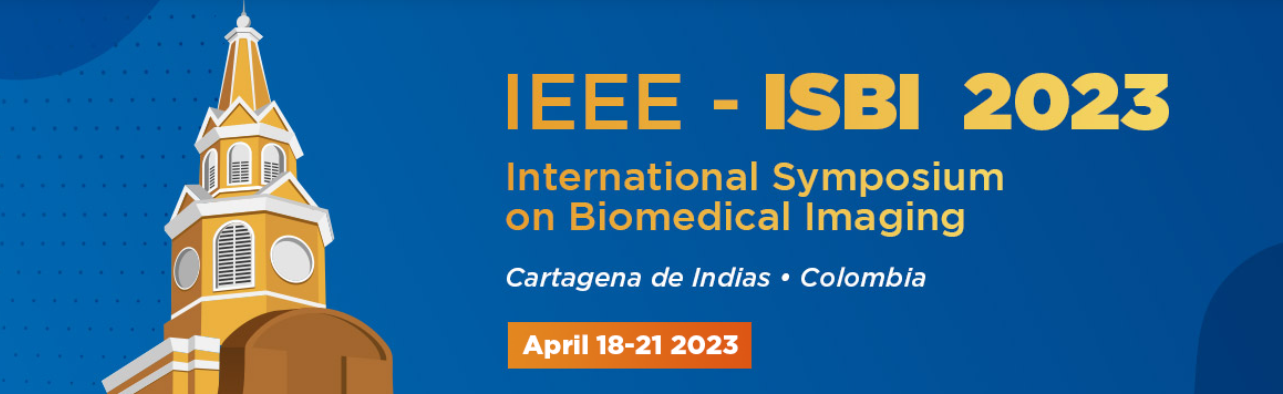
PAIP 2023 has been selected as a challenge of the IEEE International Symposium on Biomedical Imaging (ISBI 2023)
Notice
The validation submission is re-opened!! (05, Jul. 2023)
About
Participants will be required to tackle the following two tasks.- computation of TC in pancreatic cancer with tumor cell nucleus segmentation using a decent-size training dataset (images and annotations).
- calculating TC in colon cancer with tumor cell nucleus segmentation under the situation of having a tiny training dataset but having a decent training dataset for another task.
Schedule
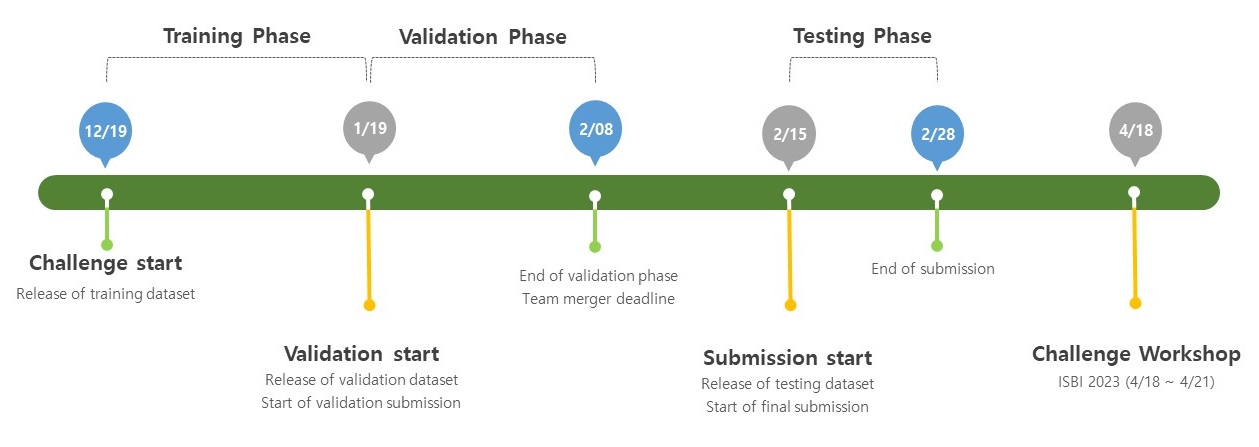
Dec. 19, 2022 ------- Training dataset released (KST 10:00)
Jan. 19, 2023 ------- Validation dataset released & submission start
Feb. 08, 2023 ------- End of submission (validation)
Feb. 15, 2023 ------- Testing dataset released & submission start (KST 10:00)
Feb. 28, 2023 ------- End of submission (test/KST16:00)
How to participate
1. Sign up for a Grand Challenge account.
(You should get ID verification. Unverified users will not be available to submit the algorithms according to the Grand Challenge policy.)
2. Read the challenge rules carefully.
3. Join the PAIP 2023 Challenge. (Click the join button.)
4. Submit a Data Use Agreement Consent form (DUA).
(You should sign up to submit the data use agreement.)
5. Download the datasets from a link on the confirmed email with access credentials.
6. Submit your result with an abstract.
If you have any questions, please contact us at paip.challenge@gmail.com.
Aims
PAIP 2023 challenge aims to promote the development of automatic and reliable TC evaluation algorithms that are flexibly applicable to multiple organs. The PAIP 2023 challenge poses two practical problems for developing automated image analysis tools for reliable TC evaluation.
Motivation
Tumor cellularity (TC) is used to compute the residual
tumor burden in several organs, such as the breast and colon. The
TC is measured based on semantic cell segmentation, which
accurately classifies and delineates individual cells. However,
manual analysis of TC is impractical in clinics because of the large
volumes of pathological images and is unreliable owing to
inconsistent TC values among pathologists. Essentially, tumor cellularity should be calculated by individual cell
counting; however, manual counting is impossible, and human pathologists cannot avoid individual differences in diagnostic performance. Automatic image analysis is the ideal method
for solving this problem, and it can efficiently reduce the workload of
pathologists.
References
[1] Akbar, Shazia, et al. "Automated and manual quantification
of tumour cellularity in digital slides for tumour burden
assessment." Scientific reports 9.1 (2019): 1-9.
[2] Akbar, Shazia, et al. "Determining tumor cellularity in
digital slides using resnet." Medical Imaging 2018: Digital
Pathology. Vol. 10581. SPIE, 2018.
[3] Wang, Pin, et al. "Automatic cell nuclei segmentation and
classification of breast cancer histopathology images." Signal
Processing 122 (2016): 1-13.
[4] Pei, Ziang, et al. "Direct cellularity estimation on breast
cancer histopathology images using transfer learning."
Computational and mathematical methods in medicine 2019
PAIP 2023: Tumor cellularity prediction in pancreatic cancer (supervised learning) and colon cancer (transfer learning)
4
(2019).
[5] Heid, Irina, et al. "Co-clinical Assessment of Tumor
Cellularity in Pancreatic Cancer ADC Predicts Survival in
Pancreatic Cancer." Clinical Cancer Research 23.6 (2017):
1461-1470.
[6] Rakhlin, Alexander, et al. "Breast tumor cellularity
assessment using deep neural networks." Proceedings of the
IEEE/CVF International Conference on Computer Vision Workshops. 2019.
PAIP platform
PAIP provides more high-quality pathology slide images of six tumor types as well as region-of-interest annotations developed by pathology experts. Please find this website http://wisepaip.org.